
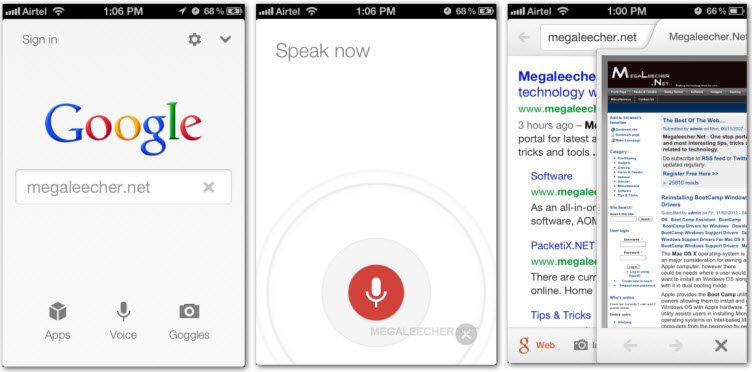

They must prioritize identifiable threats in this data to respond quickly, given that threats can have an impact within minutes. Since attackers seek to evade detection, security analysts must correlate evidence of suspicious activity across a staggering volume of inputs. Security operations (SecOps) is particularly fertile ground for innovation. The emergence of generative AI has introduced further opportunities for the application of AI to security priorities. Unsupervised approaches, meanwhile, have arisen to provide greater autonomy to security data analysis, which can offer greater alleviation of a security operations team's burden in recognizing significant events and artifacts in an often overwhelming volume of telemetry from a wide range of sources. Supervised machine learning has often been called upon to refine approaches to security analytics previously characterized by rules-based event recognition. The application of machine learning to identifying activity baselines and anomalies that stand out has spurred the rise of user and entity behavior analytics, which can often provide early recognition of malicious activity based on variations from observed norms in the behavior of people as well as technology assets. The sheer number of malware types and variants have long demanded an approach to this aspect of threat recognition that is both scalable and responsive, given the volume and rapid pace at which new attacks emerge to stay ahead of defenses.
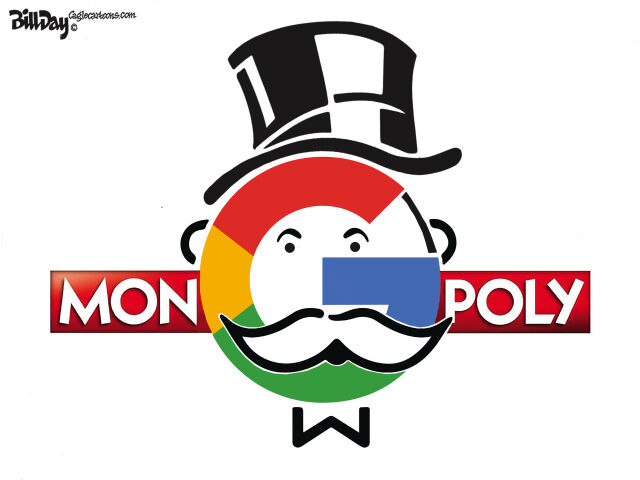
Machine learning has played a role for many years already in security efforts such as malware recognition and differentiation. Both have made a substantial mark already on the technology products and services markets. We believe that the distinctions between AI for security and security for AI help define the broad outlines of coverage we plan for both. Another 46% of respondents say security is a concern, if not a top concern, giving a total of 67% of respondents reporting some degree of concern about security - the largest percentage of any response. These two (security and cost) well outdistance the next concern, reliability (11%). In terms of security for AI, security is the most frequently reported concern about the infrastructure that hosts, or will host, AI/ML workloads (21% of respondents, ahead of cost at 19%). In terms of AI for security, threat detection is the most frequently reported area of existing investment (47% of respondents), and another 37% say they plan future investment. These include the application of AI to security issues and opportunities, which we abbreviate here as "AI for security," and the security of the implementation, deployment and use of AI, which we refer to as "security for AI."Īccording to 451 Research's Voice of the Enterprise: AI & Machine Learning, Infrastructure 2023 survey, both aspects of this intersection are prominent for respondents implementing AI/machine learning (ML) initiatives. In this report, we introduce distinctions between the intersection of these topics evident at these events. That same week, DEF CON hosted the largest public "red teaming" (penetration testing) exercise against AI models to date. It was also the theme of the opening keynote at Black Hat, where the AI Cyber Challenge, a Defense Advanced Research Projects Agency (DARPA) initiative launched by the Biden-Harris administration, was announced. As with many nascent trends, security often rises to the top of opportunities as well as concerns, and this is no less true with AI - it was a central focus of this year's RSA Conference. AI has been a trending topic in technology for many years, but nothing has fueled interest like the explosive emergence of generative AI over the past year.
